Artificial Intelligence in HDD and SSD reliability analysis: New Horizons
In today’s world, artificial intelligence (AI) is becoming an integral part of various technologies and processes. From medicine to the automotive industry, we encounter its applications every day. But have you thought about how AI can help in the area of data storage? Specifically, could it be used to analyze the reliability of hard disk drives (HDDs) and solid state drives (SSDs)? Unexpected HDD failures can cause serious problems, including server downtime and data loss. Often, HDDs don’t give a clear indication of their condition, leaving technicians to rely on uptime and experience. In this article, we look at how AI can change the approach to disk health monitoring and prevent potential failures.
Traditional diagnostic methods
These days, S.M.A.R.T.-testing (Self-Monitoring, Analysis and Reporting Technology) is still the go-to way to check the health of hard disk drives. This method involves collecting a variety of data points, such as disk uptime, read and write error rates, and the number of bad sectors. In total, the S.M.A.R.T. system tracks about 255 different attributes, though manufacturers may restrict access to some of them.

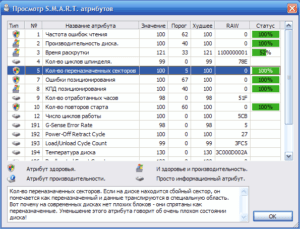
While S.M.A.R.T. is really useful, it does have its limitations. For instance, it can’t always predict when a disk will fail, especially in the case of sudden failures. Plus, technicians often have to rely on their own experience and intuition, which doesn’t always guarantee a successful solution to the problem.
Applying AI for failure prediction
A popular technical resource, Habr.com, recently published an article on using AI to analyze the reliability of HDD disks. The authors of the study came up with a new way to do this by looking at lots of historical data on disk failures.
They used data from two large companies to create the AI model:
- Data from BackBlaze: This U.S.-based company has been publishing S.M.A.R.T. diagnostics of its hard drives since 2013. They provide extensive statistics on 85 different drive models, including information on when they fail. With this data, researchers have been able to get an idea of how different drive models behave under real-world conditions.
- PAKDDD2020 Alibaba AI Ops Competition: This contest asked participants to develop a model for predicting disk drive failures based on anonymized S.M.A.R.T. data. It is important to note that the data for the competition contained information about hard drive manufacturers, but this information was hidden, making it difficult for the contestants. Despite the lack of information about specific disk vendors, the data contained enough attributes to successfully train the model.
Creating and testing an AI model
The model development process involved several steps. The first step was to collect and process historical data, including S.M.A.R.T. attributes and actual failure information. The next step was to clean and normalize the data to eliminate anomalies and ensure that the machine learning algorithms would work correctly.
Correlations between different attributes and failure times were then analyzed to identify the most significant factors. Based on this data, several machine learning models were developed and trained using different algorithms such as random forests, gradient bousting, and neural networks.
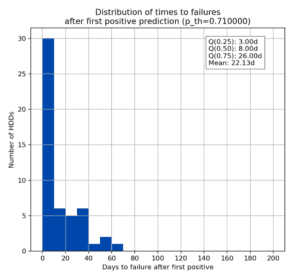
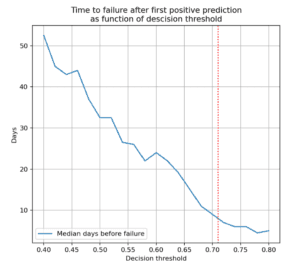
After training, the models were tested on new data to evaluate their accuracy and reliability. The results showed that the model was able to predict the probability of disk failure in the coming days with high accuracy, allowing for timely replacement of potentially unreliable devices and preventing downtime.
Model Pros:
The resulting model is quite universal – there is no critical dependency on the SMART disk data used in a particular organization. This means that it does not require a complex system of regular collection of SMART data and disk failure events. This is its main value.
Cons of the model:
It is not possible to write a disk failure prediction model that is applicable to different disk models. You will still need to train a separate model for each disk model. This is because each disk model may have a different set of SMART attributes. In addition, the wear in the SMART attributes is unique to each disk model.
Conslusion
The authors’ models can provide quite high accuracy. In some cases, Precision reaches 70%, but these models fail to predict a significant number of failures. The recall metric has never exceeded 50%, which means that half of the disks fail for reasons that the model does not understand. These failures can be called “sudden deaths”. It is likely that such a large number of sudden deaths indicates that the SMART data is simply not enough. And the fact that the winners of the Alibaba contest, recall is only 40%, confirms this hypothesis.
The use of artificial intelligence opens up new possibilities for monitoring and predicting disk drive failures. The models are already showing good results, especially in terms of prediction accuracy. However, there are still unresolved issues related to sudden failures and limited S.M.A.R.T. data.
The authors of the project continue to work on improving their models and hope that with new data it will be possible to improve the efficiency of predictions. Despite the existing difficulties, the application of AI in the field of diagnostics of hard disk drives and solid-state disks (HDD and SSD) seems to be a promising area of information technology development.
You can read more about the model, including technical nuances (all sorts of different parameters of the AI model), in the original article at Habr.com.
You might find these blog posts interesting:
Artificial Intelligence in HDD and SSD reliability analysis: New Horizons
Artificial Intelligence in HDD and SSD reliability analysis: New Horizons In today’s world, artificial intelligence…
What Google Doesn’t Know About HDD Temperature That You Should
Studies conducted by Google and Backblaze in the same breath conclude that “As long as…